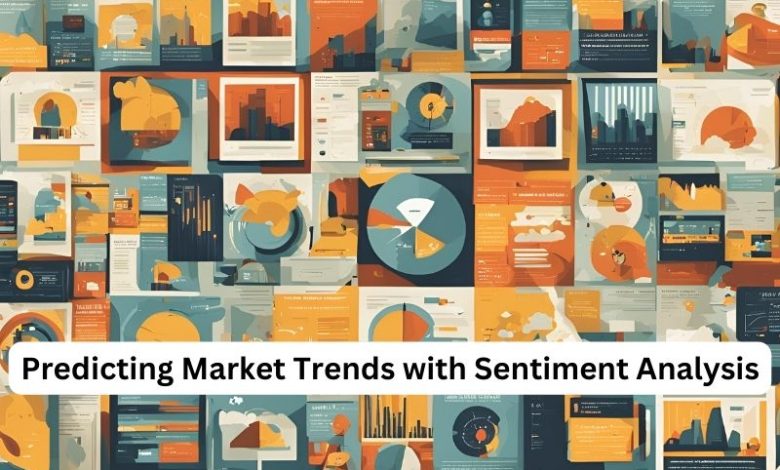
Predicting Market Trends with Sentiment Analysis
Predicting market trends using sentiment analysis is a cutting-edge application of data science that leverages the vast amounts of unstructured data generated from social media, news articles, financial reports, and other digital communications. Sentiment analysis involves using natural language processing (NLP), text analysis, and computational linguistics to identify and extract subjective information from text. This technique allows businesses and investors to understand public sentiment toward products, services, companies, or markets, which can be crucial for making informed financial decisions. A Data Scientist Course in Hyderabad, Bangalore, Mumbai, and such cities where businesses rely on technologies to capture market space and generate revenue finds large-scale enrolments from business professionals, especially if the course covers marketing disciplines such as sentiment analysis.
Sensing Market Trends with Sentiment Analysis
Here is how sentiment analysis is used to predict market trends:
The process of sentiment analysis
1. Data Collection
The first step involves gathering data from various sources. This includes social media platforms like Twitter and Facebook, financial news websites, blogs, forums, and other online platforms where people express their opinions. The quality and relevance of data collected are critical, as they form the foundation for accurate sentiment analysis.
2. Preprocessing and Cleaning
The collected data often contains noise such as irrelevant posts, advertisements, and spam. Data preprocessing includes cleaning the data, removing irrelevant content, and standardizing the format to ensure the analysis is performed on relevant and high-quality data. While these are basic steps that will be covered in detail in any entry-level Data Science Course, these are also the most crucial steps that ultimately decide the accuracy and reliability of the inferences derived using data analysis.
3. Sentiment Extraction
Using NLP techniques, sentiment analysis algorithms classify the polarity of the text as positive, negative, or neutral. More advanced models might also measure the intensity of the sentiment. This step typically involves techniques such as tokenization, stemming, and lemmatization, followed by the application of sentiment scoring methods which might be based on predefined lexicons or machine learning models. These techniques are part of the curriculum of any mid-level or advanced-level
4. Integration with Quantitative Data
Sentiment data is often combined with traditional quantitative market data such as stock prices, trading volumes, and economic indicators. This integration allows analysts to see a more complete picture of market conditions. For instance, a sudden spike in negative sentiment on social media regarding a company could be a precursor to a drop in its stock price.
5. Trend Prediction Models
Machine learning models can then be used to correlate sentiment scores with market trends. These models can identify patterns that precede market movements. Common algorithms used include regression models, decision trees, and neural networks. Time-series analysis might also be applied to track sentiment over time and its impact on market trends.
6. Real-time Analysis and Decision-Making
In today’s fast-paced market environment, the ability to analyze sentiment in real time can provide a significant edge. Real-time sentiment analysis tools help traders and analysts make quicker decisions by providing up-to-date insights into public opinion and market trends.
7. Risk Management
By understanding market sentiment, companies, and investors can better manage risk. If sentiment analysis indicates a potential negative shift in market perception, a firm might decide to postpone a product launch or a public offering, thus avoiding potential losses.
8. Feedback Loop
Implementing a feedback system to assess the accuracy of predictions and the effectiveness of trading strategies based on sentiment analysis is crucial. This feedback helps in refining models and strategies over time, improving the accuracy and reliability of predictions.
Challenges and Considerations
An inclusive data science course must also cover the challenges faced and the considerations that have evolved in sentiment analysis. A few of the more common ones are listed here.
Bias and Noise: Social media and other platforms often contain biased opinions and irrelevant information, which can skew the analysis.
Sarcasm and Context: Detecting sarcasm, irony, and context accurately is a challenge for current NLP models.
Dynamic Nature of Language: The way people express sentiment can change rapidly, requiring continuous updates to models and lexicons.
Conclusion
Predicting market trends with sentiment analysis is a powerful example of how data science can extract actionable insights from unstructured data, helping businesses navigate complex market dynamics and make data-driven decisions. Most businesses in commercialized cities rely predominantly on sentiment analysis to evolve effective, customer-facing marketing strategies. Thus, a business professional who has completed a Data Scientist Course in Hyderabad that covers sentiment analysis is one of the most sought-after professionals in the job market.
ExcelR – Data Science, Data Analytics and Business Analyst Course Training in Hyderabad
Address: Cyber Towers, PHASE-2, 5th Floor, Quadrant-2, HITEC City, Hyderabad, Telangana 500081
Phone: 096321 56744